Image Map – Visualizing Image Data with imshow
The imshow
function is used to visualize image data and is primarily designed to display two-dimensional arrays as images.
To use it, simply pass an array of image data to imshow()
. The function provides various color maps and interpolation options, allowing you to display images in different styles.
For example:
- Use the
cmap
argument to set the color map. - Use the
interpolation
argument to control how the image is rendered.
This makes imshow()
a powerful tool for visualizing heatmaps, grayscale images, and other data-based visualizations.
Pros of Using imshow
- Simplicity
- Easily visualize two-dimensional arrays as images.
- Convenient for data analysis and processing.
- Flexibility
- Offers various color maps and interpolation options to customize image representation.
- Extensibility
- Can visualize not only images but also complex data structures.
- Useful in scientific research and engineering analysis.
Key Use Cases
- Scientific Research
- Represent and analyze experimental data as images.
- Used in fields like physics, chemistry, and biology.
- Machine Learning
- Process image data and visualize results.
- For example, display feature maps from convolutional neural networks (CNNs) to understand network behavior.
- Geographic Information Systems (GIS)
- Visualize satellite imagery or terrain data.
imshow
is a powerful tool for converting numerical data into meaningful visual representations across various fields.
Image map Code
import matplotlib.image as img
import matplotlib.pyplot as plt
import numpy as np
image = img.imread('./imshow.png')
# image array
# [[[0.9372549 0.9254902 0.89411765]
# [0.91764706 0.92941177 0.89411765]
# [0.9137255 0.93333334 0.8901961 ]
# ...
plt.imshow(image, cmap='viridis')
plt.colorbar()
plt.title('Array to image')
plt.show()
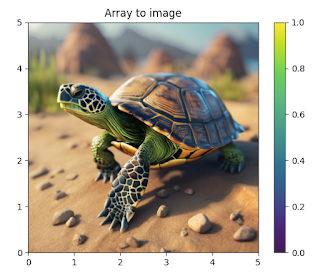
# Color Gray
plt.imshow(image[:,:,[0,0,0]], cmap='viridis')
plt.colorbar()
plt.title('Array to image')
plt.show()
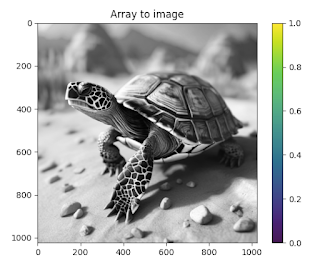
# Gray Channel
plt.imshow(image[:,:,0], cmap='gray')
plt.colorbar()
plt.title('Array to image')
plt.show()
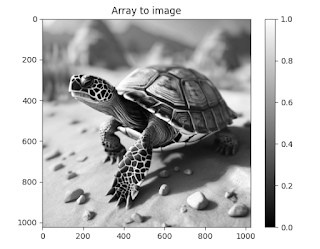
First of all, I wrote this case as an example of color modification. In reality, I needed to extract text from an image using OCR (Optical Character Recognition) to read a children’s storybook to my kids.
I had heard that preprocessing an image before OCR improves recognition accuracy, so I gave it a try. Interestingly, the older version of the OCR software could recognize the text more effectively after preprocessing.
I wonder if modern OCR technology would still benefit from preprocessing or if it would work just as well without it.
After this experience, I started paying more attention to data source quality and optimization, realizing how crucial they are for accurate results.